Artificial intelligence demonstrates power to predict and prevent LV cable failures
by Brian Lasslett, Head of Innovation & IP Management
Fundamentals is working with DNOs to roll out an innovative AI-based technology with the potential to slash the incidence and cost of LV cable power outages. It will do this by identifying and locating pre-faults with unprecedented accuracy before they cause failures.
Developed initially as an NIA (Network Innovation Allowance) project, field trials of our SYNAPS (Synchronous Analysis and Protection System) solution are ongoing in partnership with DNOs. Early results show it can identify the probability of future failure by >95% and location accuracy >3 metres – including spurs.
We believe SYNAPS has the potential to transform LV cable asset management, enabling DNOs to switch from reactive repairs when fuses rupture and cause outages to planned interventions before faults occur.
Current technology limitations
LV cable issues cause 80% of all faults in the power network at distribution level and are responsible for high levels of customer complaints and customer power outages. A disproportionate share of ongoing network costs is devoted to finding and fixing them.
Current fault-fixing technology has two drawbacks: most faults can only be identified with certainty when fuses rupture or reclosers operate – and TDR and impedance-to-fault devices have their limitations when used to pinpoint the fault locations. For example, they are intrusive devices that require a power outage to install, single-ended solutions can only identify the distance of a fault from the substation – not which spur it is on, so they identify multiple possible locations, and the distance is always given with a distance variation, typically +/- 10-20 metres.
The SYNAPS game-changer
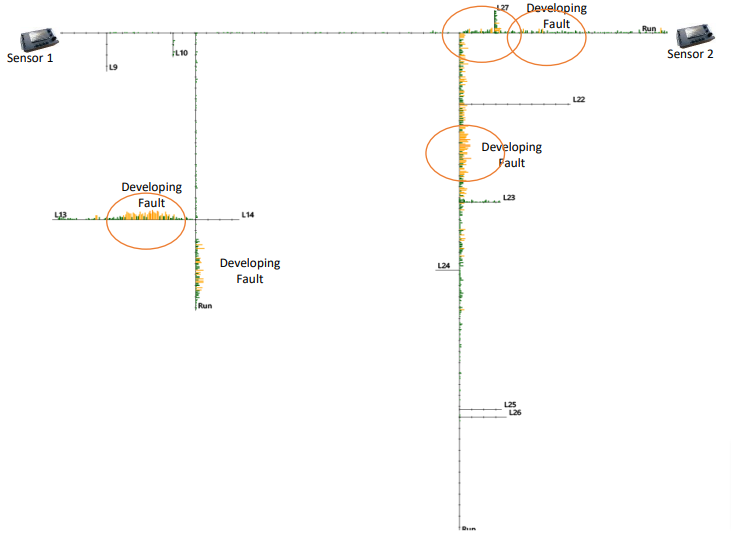
SYNAPS’s dual sensors detect and locate pre-faults on any point of the network. AI algorithms classify all the events in terms of probability of failure and location, eliminating false alarms.
SYNAPS takes a radically different approach to the issue. It uses two sensors, installed passively (requiring no power outage) on the network, to capture waveforms across the LV network. Waveforms record all activities on the network, including transient ‘pre-fault’ events. These are automatically analysed using AI algorithms which identify activities that are symptomatic of developing faults. These include water ingress, mechanical failure of joints and insulation breakdown. As well as detecting and classifying potential pre-faults, it accurately locates them at any point on the network.
SYNAPS’s ability to discriminate between significant pre-fault activity and general noise has already proved accurate in trials. The application of deep machine learning and the ability of the algorithm to improve itself by creating artificial ‘neural networks’, increase its accuracy with every use. Pre-faults are classified in terms of fault type, locations and probability of failure, so DNOs have the data to prioritise repairs and replacements, based on the risks of future outages. In other words, they have unprecedented information on when to dig and where to dig for pro-active asset management.
Proof of concept
The power of SYNAPS to avert failures, before fuse ruptures or recloser operation, has been demonstrated at a long-term asset management trial site in Portsmouth, UK.
SYNAPS sensors, which were capturing events over several months, detected the development of a phase-phase pre-fault in a joint, without it triggering a protection device or fuse rupture. Confidence in the nature and location of the event increased as monitoring continued. The decision to excavate was taken and the results, independently verified, were:
- SYNAPS identified the developing fault before it ruptured a fuse or caused a failure.
- It located the event accurately – in a Wavecon/CONSAC joint, 25m from the substation.
- There was arcing between two phases in the joint at the connection of service cables.
- Thermal cycling had caused small movements, leading to abrasion and mechanical fatigue of the paper insulation.
Ready to deploy NOW
SYNAPS has already moved from a working prototype, into an off-the-shelf product called COPPsystem (Cable Outage Prediction & Prevention). Additional trials are taking place under the NIA scheme, which will provide further evidence of its effectiveness and accuracy – and use deep machine learning to make it progressively more accurate, the more it is deployed.
We are also training DNOs’ own personnel to install, run and maintain COPPsystem, ready to roll it out as part of business as usual on their own LV networks.
The future: from LV to MV and HV
COPPsystem has proved it can report the probability of failure resulting from LV cable pre-faults. The next stages will be the capacity to deliver accurate time-to-failure predictions, together with real-time network topology mapping. In other words, across the whole network it will provide operators with a constantly updated, detailed picture of where and when LV cables are likely to fail. This will be a step change in asset management, we believe.
We also believe future iterations of COPPsystem, using SYNAPS AI technology, have tremendous potential for failure prediction and prevention in MV and HV cable networks – in particular in offshore applications, where submarine cable faults account for up to 80% of insurance claims by operators, because of the massive cost of finding and fixing them plus major revenue losses.
For example, Danish energy company Orsted A/S has found that some of its cables connected to wind farms have been damaged by scraping against rocks on the seabed and will need to spend up to DKK3 billion (£350 million) to fix them. Read more.
Other sectors where SYNAPS could be deployed include railway networks, mining and industrial applications.
Be part of the innovation
With SYNAPS AI at its heart, COPPsystem has the potential to deliver significant benefits for DNOs and customers alike – fewer outages, fewer holes and lower costs. The more it is deployed, the smarter it will become.
Learn more
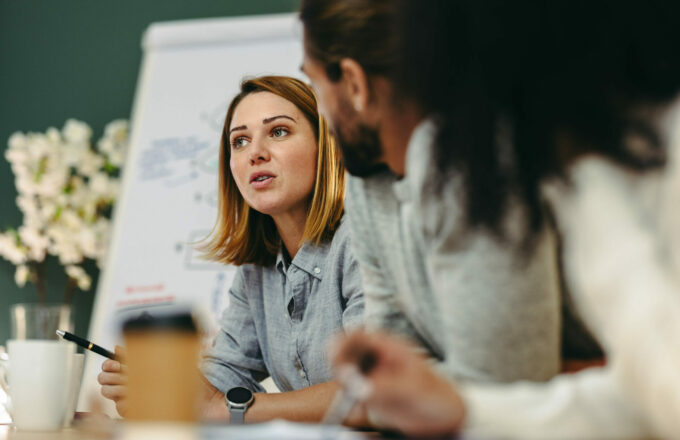
- Article
- Other
Should DNOs pay to keep their networks healthy?
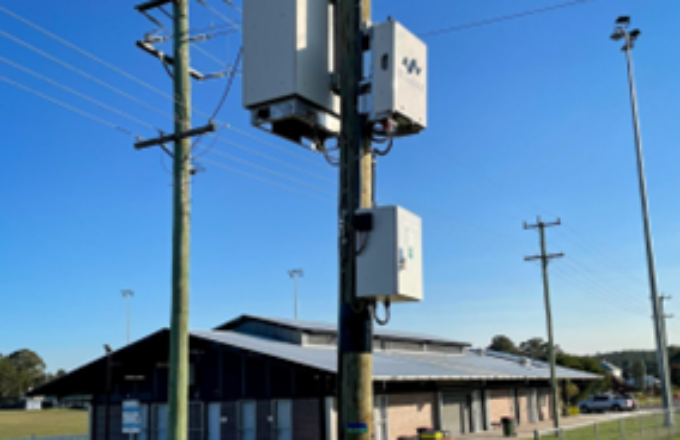
- Article
- LV Network Control
- Other
How EcoJoule solutions can tackle local EV charging issues
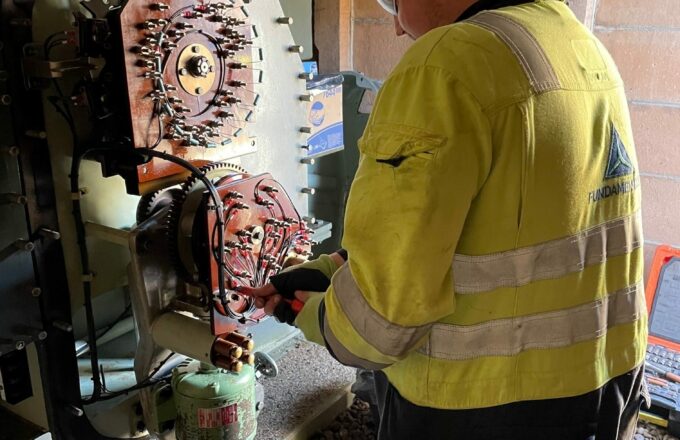
- Article
- Industry News
- Other
How apprentices can get a head start in green energy careers
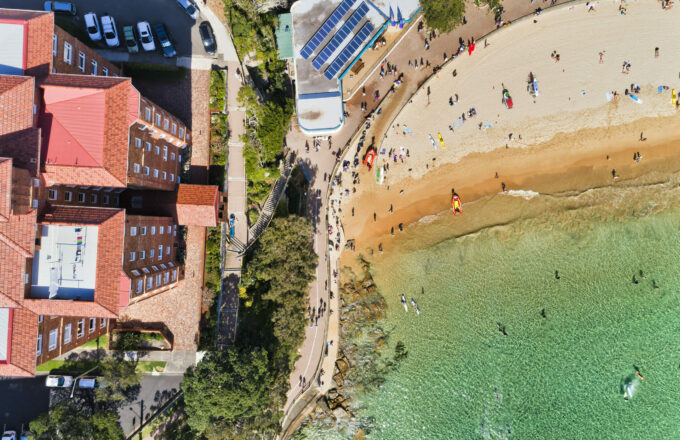
- Article
- Other